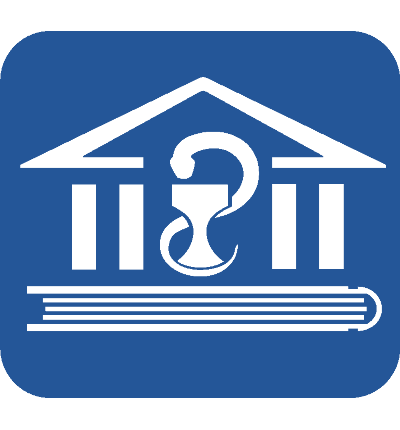
REVIEW
Ethical issues in implementing artificial intelligence in healthcare
1 First Moscow State Medical University named after I. M. Sechenov, Moscow, Russia
2 Yaroslavl State Medical University, Yaroslavl, Russia
Correspondence should be addressed: Konstantin A. Koshechkin
Nikitsky Boulevard, 13/1, of. 504, Moscow, 119991, Russia; ur.vonehces.ffats@a_k_nikhcehsok
Integration of artificial intelligence (AI) in healthcare is rapidly expanding making a revolution in various aspects of the industry. AI includes a number of technologies such as machine learning, natural language processing [1] and robotics, which are used to improve medical aid and treatment outcomes of patients [2] and optimize effectiveness of the organizational activity.
In medical diagnostics, AI-based systems can analyze medical images such as X-rays, magnetic resonance imaging and computed tomography with high accuracy and allow doctors to perform early detection and diagnostics of diseases. These systems can find subtle regularities and abnormalities, which can be missed by a human observer. It can eventually result in a faster and more exact diagnostics [3]. These systems also allow to decrease the load on doctors during massive screenings by separating medical images with no pathology from studies that require special attention of specialists.
Moreover, AI transforms treatment planning and personalized medicine [4] by analyzing large patient-related data sets including genetic profiles, case histories and treatment outcomes. Machine learning algorithms can find correlations and regularities related to the data. This helps to predict patient’s reactions to different methods of treatment and adapt interventions respectively [5].
Apart from diagnostics and treatment, AI is used to monitor patients and provide remote care. AI-equipped wearables can continuously collect and analyze physiological data, which allows to detect health-related data at an early stage and take prevention measures. AI-based telemedicine platforms make consultations and remote monitoring easier by improving access to medical services, especially in the areas with low service level [6, 7].
Moreover, AI-based systems of healthcare management optimize administrative tasks, distribute resources better and improve the processes of decision taking. The systems can analyze large data sets taken from e-cards, billing systems and administrative data bases to find tendencies, inefficiencies and room for improvement [8, 9].
Though AI integration into healthcare has great prospects, it also raises essential ethical, legal and social issues. Ensuring confidentiality, transparency of algorithms and responsibility for treatment of patients during development and implementation of AI-based technologies is important during implementation of the entire potential of these innovations while preserving trust and integrity of healthcare systems [10–12].
In a fast-evolving employment landscape of healthcare technologies, AI opens up huge perspectives for a revolution in care, diagnostics, treatment and research. However, potential advantages are accompanied by essential ethical considerations which should be properly taken into account to ensure reliable development and implementation of AI technologies.
The basic ethical imperative in healthcare includes the priority in patient’s welfare and safety. AI technologies can improve treatment outcome of patients. They also create new risks such as algorithmic bias, violated confidentiality of data and mistakes while taking decisions. When the AI systems are developed and implemented, we need to take into account some ethical considerations to reduce harm and improve benefit for patients [13].
AI can improve access to healthcare expanding coverage of medical knowledge and resources. However, there exists a risk that AI-based decisions can make the existing disproportions worse if they are not thoughtfully applied. The ethical frames should include the issues of inclusivity and accessibility. Then it will be warranted that AI is useful for all patients irrespective of their social and economic status, geographical position and other factors [7].
AI ethical development requires algorithms to work properly and explanation of results used while creating and implementing AI-based systems during the entire technology life cycle. It includes transparency of taking algorithmic decisions, sources and use of data, and the possibility to comprehend and dispute AI-based recommendations. Moreover, clear accountability mechanisms are required to eliminate mistakes, prejudices and unforeseen consequences, which can occur as a result of AI deployment [14].
Autonomy and informed consent: respect for a patient’s autonomy and right to take reasonable decisions about medical intervention belong to basic ethical principles. As AI technologies are more integrated into the clinical practice, patients should be clearly aware of how AI is used in treatment and be able to provide informed consent. This includes transparency of limitations and uncertainties of AI systems and involvement of medical workers in decision taking.
Professional integrity and trust: medical workers should act for the benefit of patient’s interests and apply ethical standards to practice. AI integration should add to experience and judgements of suppliers of medical services but not replace them. Ethical principles should support an exact use of AI as a tool to improve the process of taking clinical decisions, increase effectiveness of working processes and improve outcomes of treatment by preserving trust and integrity of relations between a patient and a supplier of medical services.
While AI continues to penetrate into various aspects of healthcare (from diagnostics and treatment to administrative tasks and interaction with patients), it gives birth to many ethical considerations that need to be examined properly. A landscape of ethical issues, which are associated with AI in healthcare, is described in the article. Ethical consequences of using AI in healthcare have been studied: from dangers related to confidentiality of patents and safety of data to potential algorithmic prejudice and discrimination, issues about accountability of AI systems while taking clinical decisions, risk of undermining confidence between patients and doctors in case of automated interventions and ethical responsibility of medical workers [12].
MATERIALS AND METHODS
The existing literature related to AI integration into healthcare has been reviewed. It included scientific journals, materials of conferences, other available literature sources and respective reports of regulating authorities and professional companies. Search inquiries included combinations of some key words such as ‘artificial intellect’, ‘machine learning’, ‘healthcare’, ‘ethics’, ‘transparency’, ‘accountability’ and ‘regulation’.
Certain cases and incidents when AI technologies resulted in ethical dilemmas in medical institutions have been found and analyzed. Criteria of case selection included relevance, variety of ethical issues and availability of similar data. Thematic reviews were taken from the published literature, news articles and recorded legal or regulatory cases.
Regulatory and ethical frameworks dealing with development and implementation of AI algorithms in healthcare have been reviewed. They include respective laws, standard regulations and ethical principles issued by respective state bodies, professional communities and international organizations. Key regulating documents include materials published by the Federal Food and Drug Administration of the USA (FDA) [15], European Union General Regulation on Data Protection (GDPR) [16], Medical Ethics Code of American Medical Association (AMA) and IEEE Global Initiative related to Ethical Aspects of Artificial Intelligence and Autonomous Systems [17].
Data collection included collection of data from various sources including scientific articles, news, political documents and legal precedents. The data were analyzed using qualitative methods including thematic analysis, finding common topics, ethical dilemmas and regulatory issues associated with AI integration in healthcare. Thematic studies were analyzed with a case study to examine certain ethical issues, involved parties concerned and results for every case.
Results of literature review, thematic studies and statutory and regulatory analysis were generalized to present an extensive review of AI-related ethical problems in healthcare. Ethical consequences, regulation gaps and strategies contributing to transparency and accountability were discussed in the light of research results and existing literature. Recommendations for politicians, suppliers of medical services and other interested parties were suggested on the basis of the results and research analysis.
Limitations of the study such as potential systematic mistakes in the selected literature and thematic studies were accepted. Efforts were taken to mitigate bias by inclusion of various points of view and sources of information. The study was mainly concentrated on ethical aspects associated with AI in healthcare without dealing with technical aspects of AI algorithms or the problem of implementation. Materials and methods used during this research were aimed at the proper and systematical analysis of ethical issues associated with AI in healthcare and at the development of strategies that help to solve the problems in practice.
RESULTS AND DISCUSSION
Lack of transparency and accountability
Lack of transparency and accountability in AI algorithms belongs to one of the most pressing ethical issues associated with AI integration in healthcare. As AI systems become more complex and autonomous, the comprehension of how the algorithms take the decisions becomes crucial to ensure justice, equality and safety of patients.
AI algorithms commonly function as ‘black boxes’. It means that their processes of decision taking are not transparent and complex for interpretation or proper examination. Lack of transparency creates significant problems both for suppliers of medical services and regulatory bodies and patients as it prevents from assessing reliability, exactness and potential prejudice which are typical of AI-based recommendations. Concerns about algorithmic prejudice, discrimination and unjust attitude are obvious in healthcare where solutions have serious consequences for treatment outcomes and welfare of patients. AI systems trained based on biased and incomplete data can eternalize and exacerbate the existing inequality in rendering medical aid for certain groups of population. Moreover, non-transparency of AI algorithms makes it difficult to detect and eliminate prejudice and discrimination cases. In the lack of transparency, it is difficult to establish whether AI-based recommendations influence such factors as nationality, gender, social and economic status or other sensitive characteristics [18].
Lack of accountability further exacerbates the ethical issues as it is commonly not clear who is responsible for the actions and solutions of AI-systems in medical institutions. When AI algorithms result in erroneous or harmful results, determination of liability can be associated with legal and ethical difficulties.
To solve the issues, it is extremely important to give priority to transparency and accountability while developing and implementing AI algorithms in healthcare. This promotes open access to algorithmic methodologies and sources of data, creating conditions for independent audit and validation of AI systems, development of clear mechanisms of asking for medical aid and recovery of damage in case of algorithmic errors or causing harm.
The issues of confidentiality and safety of patient’s data
In the era of digital healthcare, when large patient-related data sets are generated, collected and analyzed, concerns about data confidentiality and safety become more and more serious. AI integration in healthcare exacerbates the problems because AI systems significantly depend on access to large data sets to train algorithms and take reasonable decisions. However, collection, storage and exchange of confidential medical data are connected with significant ethical issues, which should be decided to protect confidentiality of patients and data safety.
One of the main problems includes the possibility of an unauthorized access to medical data whether as a result of cyber-attacks, data leakage or unauthorized disclosure. Unauthorized access to confidential medical data does not only threaten personal privacy but also poses risks to safety. Patients can expect that their medical data will be handled in a responsible way and that violation of trust can have remote consequences both for people and for medical organizations.
Moreover, distribution of interrelated healthcare systems and data exchange between platforms and institutions raise additional concerns about functional compatibility and data control. Patients can be informed not well enough and have poor control over how their data are collected, transferred and used. This makes them feel that they are vulnerable and that they lost their autonomy. Moreover, aggregation of separated data sets to educate AI can unintentionally disclose confidential information or promote repeated identification of people. This creates risks for inviolability of private life and confidentiality of patients [19].
Apart from external threats, internal risks (improper use of data, unauthorized access of medical personnel and unauthorized data leakage) are worth of attention, too. Suppliers of medical services and organizations should implement reliable systems of data management, means of access control, deciphering mechanisms and audit protocols. Then they will reduce these risks and ensure safe treatment of medical data of patients during the entire life cycle. Ethical considerations associated with confidentiality of patients and safety of data are beyond compliance with regulatory requirements and cover wider principles of respecting autonomy, confidentiality and confidence. Patients should be able to take reasonable decisions related to collection, use and exchange of medical data. Suppliers of medical services should follow the highest standards of safety and confidentiality of data [17].
As soon as electronic medical cards (EMC) and portable devices of health monitoring appeared, huge sets of confidential data are collected on a regular basis. They include personal identifiers, case history, diagnostic test results, treatment plans, etc. [19].
Collection of the comprehensive body of data raises concerns about unauthorized access, unauthorized use or operation, especially when the data are not properly anonymized.
Data storage and safety
Storage of confidential medical data in digital format creates vulnerabilities for cyber-attacks, data leakage and unauthorized access. Healthcare institutions should invest into reliable measures of data safety including encrypting, access control, firewalls, and intrusion detection systems to protect patient’s data from intruders. Exchange of health-related data among suppliers of medical services, researchers, insurance companies and other organizations is essential for treatment coordination, conduction of studies and facilitating the exchange of data. However, it poses risks to personal privacy and confidentiality of patients. Inadequate data exchange protocols, weak mechanisms of authentication and insufficient measures of data protection can result in unauthorized data disclosure, breach in confidentiality and potential harm for patients.
Healthcare organizations should be guided by regulatory requirements concerning collection, storage and exchange of confidential medical information. They include such laws as Health Insurance Portability and Accountability Act (HIPAA) in the USA, General Data Protection Regulation (GDPR) in the EU, and Federal Law ‘On Personal Data’ as of 27.07.2006 № 152-FZ describing strict safety measures to protect confidentiality of patients and data safety [16, 20].
Non-compliance with these data can result in serious fines, reputation damage and loss of patient trust. Ethical considerations go beyond simple compliance with regulatory acts and cover wider principles of harmlessness, fairness and respect for patients’ rights.
Potential erosion of confidence between a patient and a doctor
In medical institutions, patient-doctor relationship is characterized by confidence, empathy and joint decision taking. Nevertheless, growing AI integration in healthcare introduces a new dynamic that can undermine the confidence and worsen attitudes between a patient and supplier of medical services. Patients often attach great importance to human communication, which they share with medical workers.
Introduction of decision process taking and AI-based automatization can be perceived as replacement of human interaction with depersonalized technologies leading to the feelings of alienation, division and distrust.
The patients can be scared that AI systems lack empathy, comprehension and intuition, which is typical of medical workers. The fact reduced the quality of medical aid and experience of patients. Patients can feel uncomfortable because they trust their medical solutions to AI systems. The systems do not totally comprehend what raises concerns about transparency, accountability and possibility of errors or prejudice.
Patients also value autonomy and ability to actively participate in taking health-related decisions. Growing dependence on taking decisions based on AI and automatization systems can weaken patient’s feeling of control over treatment and make them feel disenfranchised and marginalized. Patients can worry that AI systems can prioritize effectiveness and efficiency but not individual preferences. This will result in solutions that won’t be in line with personal goals or preferences. Wide implementation of AI technologies in healthcare can question the authority and experience of doctors especially if AI systems are perceived as more perfect or more exact as compared to human doctors [21].
Patients can doubt the value of a doctor’s contribution and search for confirmation or second opinion from several sources including AI based systems. This can undermine the authority and confidence in suppliers of medical services. Support of autonomy, confidentiality and confidence of a patient requires suppliers of medical services to comply with a delicate balance between the use of AI technologies and preservation of basic human elements in the relations between patients and supplier of medical services.
Legal and regulatory uncertainty associated with responsibility in case of AI associated errors or harming patients
AI integration in healthcare creates new legal and regulatory problems associated with responsibility in those cases when AI systems promote mistakes or harm patients. When AI technologies are becoming more autonomous and common in the clinical setting, clarifying the legal framework governing accountability is essential to protect patient’s rights, ensure justice, and build confidence in AI-based healthcare [22].
AI algorithms commonly act as complex and dynamic systems which are developed owning to education and adaptation. Complex nature of these algorithms may hamper connection of errors or unfavorable results with certain actions or decisions. It becomes more difficult to define responsibility.
Traditional legal basis can hardly take into account peculiarities of AI technologies. This will result in uncertainty about distribution of responsibility between developers of software components, manufacturers of AI-based equipment, suppliers of medical services and other involved parties. Definition of who is responsible for errors or harm resulted from the use of AI is a doubtful issue, which does not have a clear precedent or guideline in many jurisdictions. Questions arise as to where the developer or manufacturer of the AI system, supplier of medical services who is using the technology or all of them can be responsible.
The level of human involvement and control in the process of AI-based decision taken makes distribution of liability even more complicated. Suppliers of medical services can assert that they were acting in accordance with the established protocols and recommendations, whereas developers can assert that their algorithms were used properly and in an honest manner. Establishing standards of rendering medical aid and due diligence during development, implementation and use of AI technologies in healthcare is essential to reduce risks and ensure safety of patients. However, defining the standards in the context of rapidly developing AI systems is a serious issue [22].
Suppliers of medical services can stick to various standards of rendering medical aid depending on the level of their acquaintance with AI technologies, education and access to resources. Similarly, developers and manufacturers are expected to comply with the best industrial practices and quality measures to minimize the risk of mistakes or harm resulting from the use of AI.
Legal and ethical consequences: legal and regulatory uncertainty associated with the responsibility occurring in case of errors or harm associated with AI. It has deep ethical consequences for safety, justice and accountability of patients. Patients have a right to demand indemnification and compensation for harm inflicted by AI technologies. Nevertheless, lack of clear legal standards can prevent from asking for help. Removal of these uncertainties requires cooperation between legal experts, suppliers of medical services, AI developers and those who defend the rights of patients to develop complex mechanisms that balance innovations and protection of patients. They also support the principles of ethical medical practice.
AI influence on medical workers, including their change in roles, obligations and professional autonomy
Integration of AI in healthcare changes roles, obligations and professional autonomy of medical workers creating both possibilities and problems while helping patients. AI technologies expand and transform the roles of medical workers in different areas including diagnostics, treatment planning, data analysis and administrative tasks.
Suppliers of medical services are increasingly cooperating with AI systems by using their abilities to improve the process of taking clinical decisions, increase effectiveness of working processes and optimize distribution of resources.
For instance, radiologists can use AI algorithms to interpret medical images, primary care physicians can use the tools that support taking clinical AI-based decisions to provide treatment recommendations. Nurses can rely upon
AI-based chat bots to educate and support patients. AI can optimize the working processes in healthcare, reduce the administrative load and increase exactness and validity of clinical tasks. By automatizing routine tasks and using data-based analytics, medical workers can concentrate on more complicated and useful types of activity. AI based predictive analytics can detect patients with high risk of adverse events. They allow suppliers of medical services to intervene into and personalize treatment plans based on individual characteristics and requirements of patients.
Though AI technologies offer significant advantages as far as effectiveness and accuracy go, they also raise concerns about erosion of professional autonomy and decision-making power among medical workers. Suppliers of medical services may fear that AI systems will take control, especially when the algorithms act as ‘black boxes’ with non-transparent processes of decision taking. Support of professional autonomy requires to comply with a delicate balance between using AI as a tool to improve clinical practice and preserve experience, judgements and discretion of medical workers.
Examples. Case or incidence studies when AI technologies caused ethical dilemmas in medical institutions.
Algorithmic bias in diagnostic tools
Case: according to the study, the AI-based diagnostic tool used in dermatology shows the racial bias as it was less accurate while detecting skin diseases among patients with a darker skin color as compared with fair-skinned patients.
Ethical dilemma: algorithmic bias has raised concerns about unequal access to medical aid and its outcomes as patients from racial and ethnic minority groups cannot obtain sufficient help due to inaccuracies in AI-based diagnostic tools. To eliminate the bias, transparent and inclusive data collection, algorithmic audit and constant assessment are in need to ensure just provision of medical services [23, 24].
Incorrect treatment recommendations
Case: AI-based clinical decision support system recommends a high-risk surgical procedure based on incomplete or inaccurate data. This leads to unnecessary complications and unfavorable outcomes.
Ethical dilemma: the incident stressed that it is important to ensure accuracy, reliability and clinical validity of AI-based recommendations. Suppliers of medical services came across ethical dilemmas on whether the AI-based system should be trusted or it is better to use own experience and judgement to decline potentially erroneous offers. To find the balance between advantages of supporting AI-based decisions and need in clinical discretion and accountability, clear regulatory principles, education and surveillance mechanisms are required [6].
Breach of confidentiality in predictive analytics
Case: a medical organization introduced AI-based predicative analytics to detect patients with a high risk of chronic diseases. However, a data breach was experienced. It compromised confidentiality of medical information and exposed patients to privacy risks.
Ethical dilemma: the incident raised concerns for the compromise between accurate prediction and confidentiality of patients. Suppliers of medical services came across ethical dilemmas in relation to proper use of AI-based predictive analytics to improve health outcomes while preserving confidentiality and autonomy of patients. Enhancing data security, obtaining informed consent and introducing transparent structures of data management are essential to solve the ethical issues [25].
Autonomous decision taking in intensive care units
Case: the AI controlled autonomous robotic surgical system broke down during a complex surgical procedure harming a patient. The system failure was associated with technical failures, insufficient data for education and inadequate control by a human being.
Ethical dilemma: the incident raised issues about the proper level of AI-based system autonomy in healthcare and responsibility of suppliers of medical services for safety of patients. To find a balance between the potential advantages of AI-based automatization and need in human surveillance, intervention and accountability, a reliable assessment of risks, testing protocols and surveillance by the regulatory bodies are required [26].
Strategies of transparencies and accountability in A algorithms
Designing the AI algorithms with an open-source code allows to obtain better transparency by making the source code accessible to the public. This allows researchers, developers and medical workers properly examine algorithms, comprehend their internal working and detect potential prejudices or disadvantages. Development with an open-source code promotes cooperation, expert assessment and exchange of knowledge. This results in more reliable and responsible AI-based decisions. Promoting transparency and inclusiveness, initiatives with an open-source code can increase public confidence in AI technologies.
Audit of algorithms includes a systematic assessment of AI algorithms to assess their productivity, reliability, justice, and ethical consequences. Audit can also include examination of training data, assessment of model accuracy, testing for bias or discrimination, assessment of how algorithmic decisions influence various involved parties. Independent audit carried out by third companies or regulating authorities can provide objective assessment of AI algorithms by helping to detect and reduce potential risks and ensuring accountability of developers and users.
Development of XAI or Explainable AI makes it possible for AI to submit transparent explanations to its decisions and prognoses. XAI methods are aimed to dispel the myths about complex AI models and make it easier for people to understand how they speculate. By improving interpretability and explanation, XAI promotes confidence, accountability and taking AI-based decisions at medical institutions. Patients, suppliers of medical services and regulating bodies can better understand and properly examine AI recommendations. Then they will be able to take more reasonable decisions and improve treatment outcomes [24].
To make AI algorithms more transparent and accountable, transparent methods of dealing with data and reliable systems of data management are required. They include recording data sources, methods of data collection, methods of preliminary data treatment and policies of data use. Transparent data management methods allow involved parties to assess quality, relevance and representativity of training data used to develop AI algorithms. Implementing measures to manage data (anonymization of data, data minimization and data access control) helps to protect confidentiality of patients and reduce risks of unauthorized or improper use of data.
Regulatory surveillance and standards
Establishing regulating surveillance and standards for AI algorithms in healthcare is essential for transparency, accountability and compliance of ethical principles and legislation requirements. Regulating authorities can develop guidelines, regulations and certification processes to regulate development, deployment and use of AI technologies in healthcare [17]. For instance, over 10 standards for AI in healthcare were developed in the Russian Federation. Standards (GOST R 59921.8–2022 and GOST R 59921.9–2022) establishing general requirements to AI systems in medicine and systems of quality management entered into force in Russia on January 1, 2023.
Surveillance on the part of regulating bodies makes us sure that AI algorithms correspond to minimum standards of quality, safety and manufacture by building trust between patients, suppliers of medical services and politicians. Compliance with regulatory requirements allows to reduce risks, ensure safety of patients and comply with ethical standards in healthcare on the basis of AI.
CONCLUSION
AI integration in healthcare opens great prospects in transformation of care for patients, increased accuracy of diagnostics and rendering improved medical aid. But as soon as AI technologies are becoming more common in clinical practice, they also give rise to complex ethical issues, which should be solved as soon as possible to ensure reliable and justifiable rendering of medical aid. Transparency and accountability are especially important during development and implementation of AI algorithms. Such strategies as development with an open-source code, audit of algorithms and explainable AI can increase trust, reliability and ethics of taking AI-based solutions in healthcare. Regulating bodies, legislative bodies, suppliers of medical services and other involved parties play a key role in the development of guidelines, standards and frames regulating ethical use of AI in healthcare. In spite of problems and complex issues related to AI integration in healthcare, AI technologies produce a positive effect on treatment outcomes of patients, better healthcare effectiveness and stimulation of innovations. When the involved parties stick to ethical principles, ensure transparency and accountability and pay primary attention to welfare of patients, they can utilize the modifying AI potential and support the highest standards of ethical medical practice. Solution of ethical issues associated with AI in healthcare requires coordinated efforts of all involved parties to achieve the balance between innovations and ethical considerations. By solving the issues jointly and in a responsible way, we can warrant that AI technologies will ensure healthcare development preserving trust, dignity and rights both of patients and medical workers.