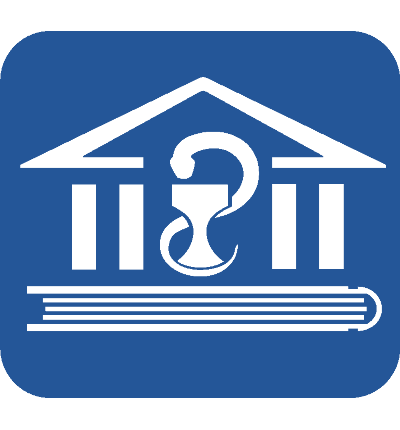
This article is an open access article distributed under the terms and conditions of the Creative Commons Attribution license (CC BY).
REVIEW
Evidence obtained during real world data analysis: what it is and how we form it
IP Pavlov First Saint Petersburg State Medical University, Saint Petersburg, Russia
Correspondence shall be addressed: Elena Vladimirovna Verbitskaya
L’va Tolstogo Str., 6–8/1, Saint Petersburg, 197022, Russia; ur.tenretni@ayakstibreve
Author contribution: Verbitskaya EV and Kolbin AS made an equal contribution to the preparation of the article.
Randomized controlled clinical trials (RCTs) are considered the gold standard for obtaining pre-marketing evidence of drug safety and efficacy. However, the life cycle of a medicinal product certainly does not end after registration. Real world data (RWD) expand and add to the knowledge [1–3]. When making regulatory decisions, real world evidence (RWE) is increasingly being taken into account. Real world evidence is clinical evidence of the use, potential benefits, or risks of medical technology application obtained from the analysis of real world data [4]. The reasons why RWE is actively gaining supporters are that classical clinical trials, especially RCTs, have a number of following significant limitations and disadvantages:
- Limited external validity. RCTs are often performed under strictly controlled conditions that may not correspond to real clinical practice, and the careful selection of study participants based on strict inclusion and non-inclusion criteria does not reflect the diversity of real patients;
- Insufficient representativeness. Many groups of patients (e.g., the elderly, those with concomitant diseases, etc.) are often excluded from RCTs, limiting applicability of the results to broader patient populations;
- Limited duration of observation. RCTs usually have a relatively short follow-up period, making it difficult to identify long-term effects and adverse events;
- High cost and complexity. Conducting RCTs requires significant financial and time costs, and complexity of design and strict protocols can make it difficult to recruit participants and complete the study.
In addition to the above, evidence based on traditional clinical studies can also be significantly influenced by ethical issues, conflicts of interest, and publication bias.
In this regard, there is a growing need in the medical community for information about the effectiveness of medicinal products (MPs) in the context of RWD. This approach allows us to assess to what extent the results obtained under controlled conditions of RCTs correspond to the effects of MPs when used in everyday medical practice. The real world evidence can be used:
- in drug development and registration;
- in studying post-registration safety and efficacy;
- in formulating clinical guidelines;
- in health economics, reimbursement systems, and pharmaceutical provision.
At the same time, RWD complements but does not replace RCT data; they are especially important for evaluation of innovative drugs and treatment of rare diseases.
In this way, RWE provides valuable information about the application of medical technologies in real clinical practice, which helps to take more informed decisions in healthcare.
There have been significant changes in RWE regulation over the past ten years. Thus, there are significant positive developments in the documents of the European Medicines Agency (EMA) and the US Food and Drug Administration (FDA) [5, 6].
Despite the growing recognition of RWE potential, an analysis published in 2021, revealed a significant gap in this area represented by the lack of comprehensive guidelines on best practices for conducting such research [7]. The authors of this analysis developed a set of criteria to assess credibility of evidence in recommendations and conducted a classification of 41 published methodological guidelines from various organizations, including regulatory authorities, health technology assessment (HTA) agencies and professional communities.
In the absence of a single standardized guideline, researchers working with the RWD are forced to rely on a disparate set of recommendations from various sources. These sources include regulatory authorities (e.g., the FDA), UK HTS agencies (such as NICE (National Institute for Health and Care Excellence)), professional organizations (e.g., ISPOR (International Society For Pharmacoeconomics and Outcomes Research)), and academic groups.
To overcome this fragmentation, it is proposed to consider the possibility of creating a systematic structure of RWE recommendations. This “organized structure” should cover all the key aspects necessary for conducting high-quality RWE research (fig. 1).
Such an approach could provide more consistent and comprehensive guidance for researchers working in the RWE field, thereby improving quality and reliability of the results obtained.
The blocks focus on the fact that high-quality RWEs are based on a fundamental scientific process that involves as follows:
- “research design’’ building block 1 includes a research plan that meets the goal and protocol development;
- “data quality” building block 2 implies the overall quality of RWD sources and identification of suitable sources for the purpose;
- “analytical methods” building block 3 includes data sources and selection of appropriate analytical methods;
- “transparency and reproducibility” building block 4 pays special attention to transparency and reproducibility when developing a research report;
- building block 5 is the final stage of the fundamental scientific process in RWD research. It gives rise to a clear understanding of how decision makers will evaluate the quality of the results obtained. This stage, known as the “final report assessment”, plays a key role in ensuring practical applicability of the study [7].
- building block 6. The main components of the RWE process are supported by two additional elements: “RWE use cases” and “demonstration projects”. “RWE use cases” define the types of hypotheses relevant to RWE studies, such as post-marketing safety assessment or expansion of drug indications.
RESEARCH DESIGN
Research planning based on data from real clinical practice is a complex process that requires a careful approach and consideration of multiple factors. A key aspect of RWE research planning is the definition of research goals and objectives. A clear formulation of the specific research tasks that need to be solved is based on what kind of evidence you want to obtain (effectiveness, safety, economic feasibility, etc.).
Figure fig. 2 presents the optimal algorithm for constructing a research design, the main initial factor of which is the purpose of the study. “Strategic/scientific considerations” are based on the purpose of the research; they determine who will use the information and how. Depending on the objectives of the study, it will be necessary to collect specific information about outcomes, ranging clinical results to patient-reported outcomes and economic results. Categories of outcomes and instruments, in turn, influence data collection needs and, ultimately, design of the study.
The “operational aspects” relate to availability of data sources and overall feasibility (what data is available, what can be easily collected, and who should be involved) and degrees of quality (what level of administrative quality and data reliability is expected by the study stakeholders). In the field of RWE, the feasibility of a study largely depends on central and local regulations, ethical and legal requirements, and the associated quality requirements of the research.
From an operational point of view, there is also a hierarchy or sequence of approaches, starting with easily accessible data from existing sources and ending with complex, long-term interventional or observational prоspective approaches to research.
If secondary data, such as claims or electronic medical records (EHR) databases, is available to achieve the purpose of the study and meet the relevant needs for results, a retrospective database study often provides a cost-effective and timely solution. To some extent, this is also true for primary research and retrospective design of medical records analysis. However, database or diagram-based retrospective approaches are limited in the possibility of follow-up or reconciliation, for example, for AE safety reports, whereas availability of information about patient-Reported Outcomes (PROs) is usually minimal.
In 2022, NICE presented generalized aspects of conducting research to collect data from real clinical practice.
By 2022, several protocol templates for RWE have been developed, most of which are based on the principles of simulating a “targeted study”, namely, the idea that first you need to write a protocol for RCT that would answer the question posed, and then translate it into an observational study protocol, taking into account possible biases that appear as randomization is lacking [8]. In 2023, an agreed HARmonized Protocol Template to Enhance Reproducibility (HARPER) of RWE research was introduced [9, 10].
The HARPER template helps understand proposed scientific solutions through a common textual, tabular, and visual structure. It contains a set of basic guidelines for clear and reproducible RWE research protocols and is intended to be used as a framework throughout the entire research process from development of a valid research protocol to registration, implementation, and reporting based on the results of this implementation [1].
In 2019, a structured tool was proposed to design pre- and post-registration comparative studies and obtain reliable and transparent real evidence (SPACE) [11].
Starting with a clear research question and following a targeted trial approach, SPACE provides a step-by-step process to identify RW research design elements and minimum criteria for feasibility and data validity issues, as well as to document research design decisions, including planned analysis. At the same time, SPACE supports the first steps in the study design necessary to identify data or compile protocol documents.
IDENTIFICATION OF SUITABLE SOURCES, HIGH-QUALITY RWD SOURCES AND SELECTION OF APPROPRIATE ANALYTICAL METHODS
Data collection and processing are key steps in research aimed at generating evidence based on real clinical practice. These processes require careful planning and organization to ensure reliability and representativeness of the data obtained.
The first step in data collection is to identify information sources. In the context of real clinical practice, such sources can include electronic medical records, patient registers, databases of medical institutions, as well as results of clinical trials and observational studies. It is important to keep in mind that data must be collected from a variety of sources to ensure their diversity and completeness. It allows to avoid systematic errors and improve reliability of conclusions.
Trust in RWD research. Data from real clinical practice are often heterogeneous and require careful preparation before analysis. In addition, for some purposes, such as calculating comparative effects, the analysis methods need to be improved. By using the data already collected, researchers can access the data before drawing up a final Statistical Analysis Plan. Data preparation and analytical decisions can have a significant impact on the final calculation results. Thus, it is necessary to eliminate the factors that can affect integrity and reliability of the evidence obtained (for example, by data dredging or a selective approach).
The data from actual clinical practice differ significantly from the data obtained during clinical trials. These differences play a key role in research planning and directly affect the quality and reliability of the RWE.
Main characteristics of RWD. The primary purpose of RWD is not originally intended for scientific purposes. They are collected to support the functioning of the healthcare system. This leads to such consequences as insufficient data ordering and possible lack of important information (for example, indications for the use of the drug or key patient characteristics in electronic medical records) [12].
Data structure. RWD can be divided into two categories:
- Structured data: ready for analysis without preprocessing;
- Unstructured data: requires identifying the structure and encoding of information. This data can be processed manually or using big data analysis and artificial intelligence technologies.
In some cases, the same information can be presented in both structured and unstructured form.
Missing data. The problem of missing data is a characteristic feature of RWD and requires special attention during analysis. Understanding these features of RWD is critically important for proper research planning, ensuring quality and reliability of the evidence obtained, and correct interpretation of the analysis results. Taking these factors into account allows researchers to develop more effective strategies of working with RWD and increase the value of evidence obtained on their basis for clinical and managerial decisions in healthcare.
Data inconsistency. Despite the existence of external controls (inspections of medical facilities by insurance companies, regulatory supervision, routine laboratory inspections), the problem of data inconsistency remains relevant. This applies both to contradictions between different sources and within the same source, especially the EMC.
Key aspects:
- External control is not a substitute for the data validation process;
- The need to identify contradictions (for example, the presence of a diagnosis in the absence of therapy or changes in laboratory parameters).
The principle of non-interference. It is important to minimize interference in the process of creating the RWD in order to avoid distortions.
Recommended approaches: implementation of checks for completeness and quality of data in source input systems, use of validation checks during data extraction, transparent documentation of all changes made and variability of data quality. The quality of RWD varies significantly depending on the source and can be unstable even within a single source.
The influencing factors are differences in how optional fields are filled in by different employees.
Recommendations for working with the RWD.
- Clearly define the prospects and volume of RWE received based on the available RWD;
- Carefully evaluate compliance of the RWD with the study objectives;
- Understand the process of creating a specific data source: who enters the information, under what conditions, based on what data, and for what purpose;
- Take into account the specifics of various sources (for example, a large number of unstructured elements and subjective assessments in the EMC);
- Use specialized guidelines for selecting RWD databases for various types of research.
Thus, working with RWD requires an integrated approach that takes into account their characteristics and limitations in order to obtain reliable and valid research results [12].
In 2021, the FDA published, as an extension of SPACE, guidelines for assessing the appropriateness of data sources for their intended purpose (Structured Process to Identify FitFor-Purpose Data (SPIFD) [11–13], which is a step-by-step process of conducting and documenting the results of a systematic feasibility assessment to ensure the suitability of data for a research question of interest. When used together, SPACE and SPIFD facilitate informed and transparent research design, planned analysis, and data selection to meet regulatory decision-making standards.
TRANSPARENCY AND REPRODUCIBILITY
The final stage of the fundamental scientific process in RWD research is formation of a clear understanding of how decision makers will evaluate the quality of the results obtained. This stage, known as the “final report assessment”, plays a key role in ensuring practical applicability of the study [7].
Compared to clinical trials and non-experimental studies that prospectively collect data, studies using routinely collected electronic medical data have greater variability in design and analysis options.
Existing guidelines and checklists have a strong consensus as to what key elements are important to communicate, but they can lead to ambiguity, assumptions, and misinterpretation when planning and implementing RWE research.
More stakeholders are moving towards routine registration of RWD studies with fully defined research implementation protocols to support regulatory and reimbursement decisions.
To increase transparency and reproducibility of the results, Wang et al. (2021) developed the structured STaRT-RWE (Structured template and reporting tool for real world evidence) template [14].
STaRT-RWE is intended for use as a didactic tool for designing and conducting qualitative RWE research; setting clear expectations for communication of RWE methods; reducing misinterpretation of insufficiently specific descriptions; enabling reviewers to quickly find key information; and facilitating reproducibility, validity assessment, and synthesis of evidence.
The template was approved by the International Society of Pharmacoepidemiology (ISPE) and the Transparency Initiative, led by the International Society for Pharmacoeconomics and Outcomes Research in partnership with ISPE, the Duke Margolis Center for Health Policy, and the National Pharmaceutical Council.
STaRT-RWE advantages:
- Improving interdisciplinary collaboration;
- Improving the quality of working with data from multiple sources;
- Optimizing the joint development and research of RWE;
- Clearer documentation of critical research details;
- Improving the effectiveness of communication between research groups;
- Improving the decision-making process based on research results.
Regulators and other decision makers encourage researchers to use standards agreed upon by the professional community when conducting and reporting on RWE research. This is necessary to obtain timely, high-quality evidence and to create a basis for evaluating and distinguishing carefully planned studies from studies with validity problems.
Thus, a structured approach such as STaRT-RWE can significantly improve the quality and reliability of real world clinical practice research, contributing to a more effective use of their results in the decision-making process in healthcare.
Adherence to essential scientific processes increases trust in research results, which can expand the range of RWE applications.
When conducting research using RWD data, it is necessary to adhere to the following key principles:
- Use of up-to-date data of appropriate quality and established origin;
- Ensuring transparency and integrity at all stages of research, from planning to reporting;
- Use of analytical methods that minimize the risk of systematic errors.
Compliance with these principles improves reliability and validity of RWE research results, which in turn increases their value for decision-making in healthcare.